
And it’s quite ‘cheap’, since it uses an existing model trained for a different purpose. It seems they’ve already done a pretty good job of filtering out most of the irrelevant/malicious sketches that were surely rampant in the raw data.īut this approach was able to easily identify a subset of sketches containing a high density of overlooked graffiti, scribbles, and irrelevant sketches. Google described their dataset as being ‘individually moderated’, and you can scroll through the examples here for a long time before hitting any graffiti (and if you do find any, you can even click it to ‘flag as inappropriate’). You can browse a gallery of all the bottom 400 flamingos here (warning: contains a few NSFW sketches).

Flamingos do bend their heads down low like that, but when asked to draw a flamingo, almost no-one thinks to pose it with its head below the horizon. The drawing above is another good example. Right: The American flamingo, illustrated by by John Audubon. God bless these artists, they really tried. We can broadly classify our worst birds into a few groups. To quote Tolstoy, happy flamingos are all alike, but every garbage flamingo is garbage in its own way.
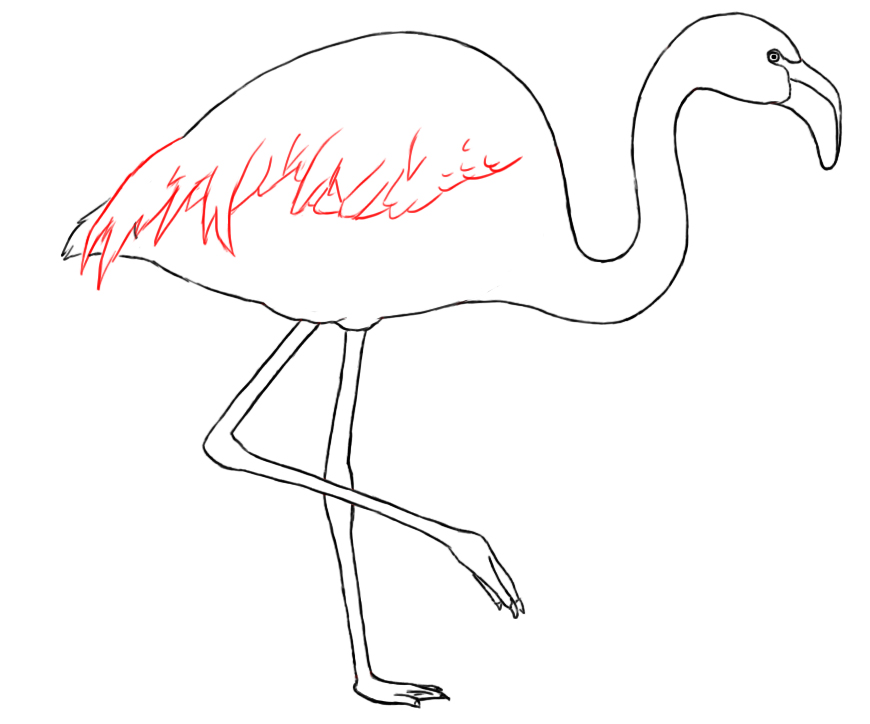
Because we’re ranking by probability, we should in some sense expect the most ‘typical’ sketches here, rather than the most beautiful. those assigned the highest probabilities:Īudubon they ain’t, but these are at least mostly recognizable as flamingos. Good flamingosīefore we roast Quickdraw’s least gifted flamingo-drawers, let’s look at some of the ‘best’ flamingo sketches - i.e. It seems reasonable that the sketches the model assigns the lowest probabilities should be among the ‘worst’. So in addition to sampling from the learned distribution to generate new sketches, we can take existing sketches, and ask the model how probable it thinks they are. As I talked about in an earlier post on character-level RBMs, learning to generate new examples of X is basically the same as learning the probability distribution of X. For example:īut we can also repurpose them to get some insight into existing human-generated sketches. These models were trained to generate new sketches. What if we want to automatically identify the jankiest flamingos in the dataset? Sketch-RNN as probability estimatorĪlong with the datasets, Google has released some pre-trained models that use the Sketch-RNN architecture described in this paper.
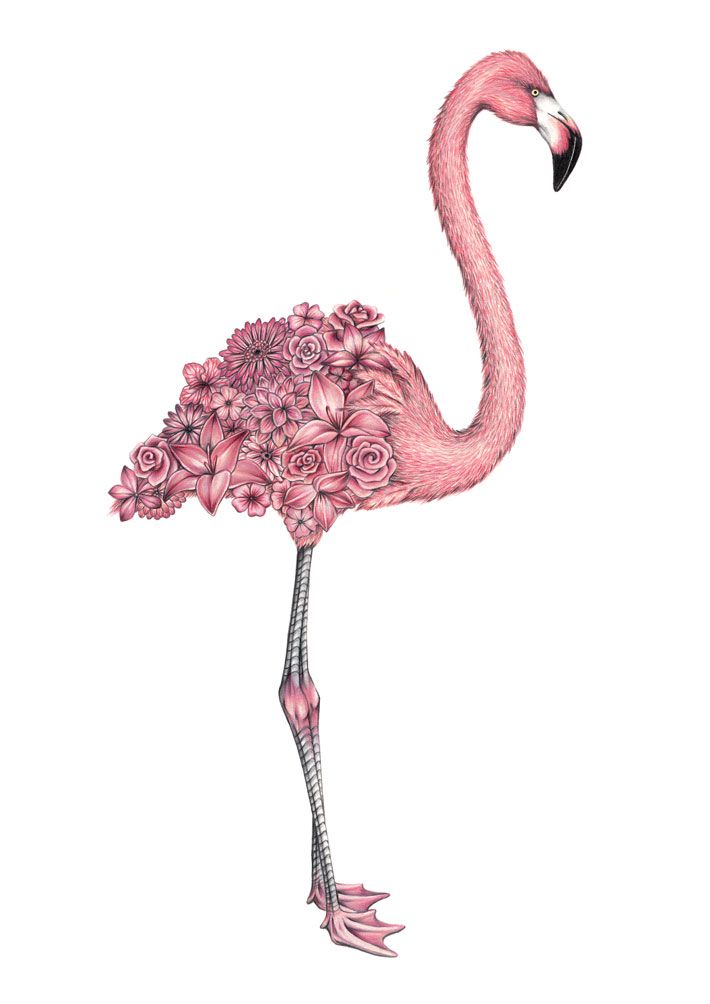
It's, um, a viking ship with a chicken carved into the prow? In this post, I’ll just be looking at sketches in the ‘flamingo’ category. Not long ago, Google released a dataset of some of the millions of sketches people have drawn so far over 345 categories. Have you played Quick, Draw! yet? It’s basically Pictionary, played against a neural network, and it’s a lot of fun.
